Cracking BING and Beyond
In Proceedings British Machine Vision Conference 2014
http://dx.doi.org/10.5244/C.28.23
Abstract
Objectness proposal is an emerging field which aims to reduce candidate object windows without missing the real ones. Under the evaluation framework of DR-#WIN, lots of methods report good performance in recent years, and the best is BING in CVPR 2014. BING provides good detection rates and surprising high efficiencies. But what can we benefit from it from the angle of computer vision research? In this paper, we show that the success of BING is rather in geometry than in computer vision research. The secret lies in the Achilles' heel of the DR-#WIN evaluation framework: the 0.5-INT-UNION criterion. We proposed a method to construct a rather small window set to ""cover"" all legal rectangles. On images no larger than 512x512, supposing all object rectangles are not smaller than 16x16, nearly 19K windows are sufficient to cover all possible rectangles. The amount is far less than that of all sliding windows. It can be reduced further by exploiting the prior distribution of the locations and sizes of true object windows in a greedy way. We also proposed a hybrid scheme blending both greedy and stochastic results. On the VOC2007 test set, it recalls 95.68% objects with 1000 proposal windows. The detection rates on the first ten windows are 13.99%~40.29% higher than earlier methods in average.
Session
Segmentation and Object Detection
Files
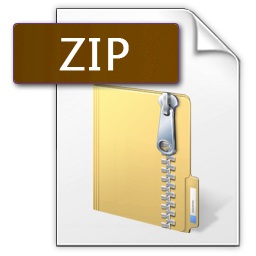

Presentation
Citation
Qiyang Zhao, Zhibin Liu and Baolin Yin. Cracking BING and Beyond. Proceedings of the British Machine Vision Conference. BMVA Press, September 2014.
BibTex
@inproceedings{BMVC.28.23 title = {Cracking BING and Beyond}, author = {Zhao, Qiyang and Liu, Zhibin and Yin, Baolin}, year = {2014}, booktitle = {Proceedings of the British Machine Vision Conference}, publisher = {BMVA Press}, editors = {Valstar, Michel and French, Andrew and Pridmore, Tony} doi = { http://dx.doi.org/10.5244/C.28.23 } }