Real-time Activity Recognition by Discerning Qualitative Relationships Between Randomly Chosen Visual Features
In Proceedings British Machine Vision Conference 2014
http://dx.doi.org/10.5244/C.28.100
Abstract
In this paper, we present a novel method to explore semantically meaningful visual information and identify the discriminative spatiotemporal relationships between them for real-time activity recognition. Our approach infers human activities using continuous egocentric videos of object manipulations in an industrial setup. In order to achieve this goal, we propose a random forest that unifies randomization, discriminative relationships mining and a Markov temporal structure. Discriminative relationships mining helps us to model relations that distinguish different activities, while randomization allows us to handle the large feature space and prevents over-fitting. The Markov temporal structure provides temporally consistent decisions. The proposed random forest uses a discriminative Markov decision tree, where every nonterminal node is a discriminative classifier and the Markov structure is applied at leaf nodes. The proposed approach outperforms the state-of-the-art methods on a new challenging dataset of assembling a pump system.
Session
Poster Session
Files
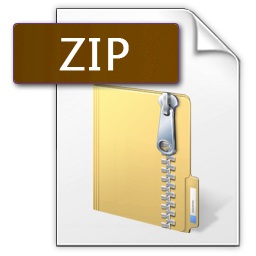

Citation
Ardhendu Behera, Anthony Cohn, and David Hogg. Real-time Activity Recognition by Discerning Qualitative Relationships Between Randomly Chosen Visual Features. Proceedings of the British Machine Vision Conference. BMVA Press, September 2014.
BibTex
@inproceedings{BMVC.28.100 title = {Real-time Activity Recognition by Discerning Qualitative Relationships Between Randomly Chosen Visual Features}, author = {Behera, Ardhendu and Cohn, Anthony and Hogg, David}, year = {2014}, booktitle = {Proceedings of the British Machine Vision Conference}, publisher = {BMVA Press}, editors = {Valstar, Michel and French, Andrew and Pridmore, Tony} doi = { http://dx.doi.org/10.5244/C.28.100 } }